Trailblazers of time series
Robert Fry Engle III and Clive William John Granger introduced innovative methods — with time varying volatility and cointegration respectively — of analysing time series data in the early 1980s
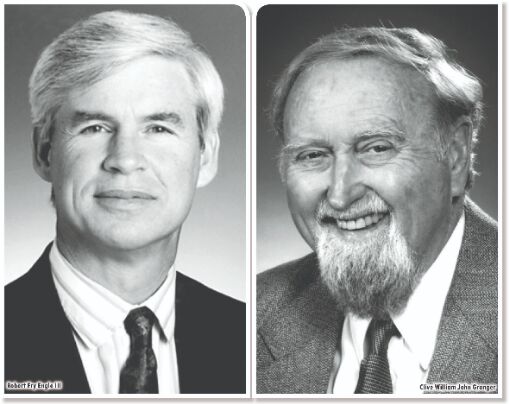
The Nobel Prize in Economic Sciences in 2003 was shared between Robert F Engle, then at New York University, "for methods of analysing economic time series with time-varying volatility (ARCH)" and Clive WJ Granger, then at University of California at San Diego, "for methods of analysing economic time series with common trends (cointegration)".
He received his Bachelor of Science degree in physics from Williams College, and his MS in physics in 1966 and PhD in economics in 1969 — both from Cornell University. After completing his PhD, Engle joined Massachusetts Institute of Technology as a faculty member and stayed there from 1969 to 1977. Thereafter, he joined the University of California, San Diego (UCSD) in 1975, wherefrom he retired in 2003. He now holds the positions of Professor Emeritus and Research Professor at UCSD. He also continues to teach at New York University at Stern School of Business. He is also the director of Volatility Institute at Stern and a co-founder of the Society for Financial Econometrics at NYU.
Granger attended the University of Nottingham for his BA, where he earned a joint degree in mathematics and economics in 1955 and a PhD in statistics in 1959 with a thesis titled 'Testing for Non-Stationarity'. He was on the faculty of the University of Nottingham until 1973, with occasional visiting positions at other universities such as Princeton. In 1973, he became a professor at the University of California at San Diego. He retired in 2003, just two months before winning the Nobel Prize. He was also knighted in 2005.
In this article we will review the main works of Engle and Granger and see how their works have been applied in various areas.
Main works of Robert Engle
Engle worked closely with Granger on concepts such as co-integration and causality, which is discussed in the section on Granger below. However, Engle was interested in the analysis of financial markets and it was here that he contributed another concept called ARCH, which is an acronym for autoregressive conditional heteroskedasticity.
Autoregressive conditional heteroskedasticity (ARCH) is a statistical model used to analyse volatility in time series. In the financial world, ARCH modelling is used to estimate risk by providing a model of volatility that more closely resembles real markets. ARCH modelling shows that periods of high volatility are followed by more high volatility and periods of low volatility are followed by more low volatility. In other words, there is a clustering of volatility and this is useful to investors when considering the risk of holding an asset over different periods of time.
ARCH models therefore did away with the earlier assumption of constant volatility and introduced varying volatility, which is how time series behave in the real world, particularly in finance markets. Engle observed that past financial data influences future data, i.e., it is autoregressive. He also knew that many financial variables such as stock market prices, interest rates, exchange rates etc. are highly volatile, but their volatility or variance is taken as constant in economic models. As noted above, Engle introduced varying volatility or variance (also called heteroskedasticity) instead of constant variance (or homoskedasticity). Another advantage of ARCH models was that they could also predict rare or 'black swan' events.
As Engle noted in his Nobel speech, he looked at ARCH not just because he observed varying volatility in financial data, but it was also a response to Friedman's conjecture that it was the uncertainty about the magnitude of inflation, rather than what the rate of inflation would be, that impacts the growth. To quote Engle:
The solution was autoregressive conditional heteroskedasticity or ARCH, a name invented by David Hendry. The ARCH model described the forecast variance in terms of current observables. Instead of using short or long sample standard deviations, the ARCH model proposed taking weighted averages of past squared forecast errors, a type of weighted variance. These weights could give more influence to recent information and less to the distant past. Clearly the ARCH model was a simple generalization of the sample variance. The big advance was that the weights could be estimated from historical data even though the true volatility was never observed. Here is how this works. Forecasts can be calculated every day or every period. By examining these forecasts for different weights, the set of weights can be found that make the forecasts closest to the variance of the next return.
ARCH models continue to evolve and have spawned others such as GARCH, EGARCH, STARCH etc. These variant models often introduce changes in terms of weighting and conditionality in order to achieve more accurate forecasting ranges. For example, EGARCH — or exponential GARCH — gives a greater weighting to negative returns in a data series as these have been shown to create more volatility.
Main works of Clive Granger
Granger was interested in applied statistics and economics from his early days, when he was a graduate student. This led him to choose time series analysis as the subject of his doctoral thesis. As part of his research, he also went to Princeton and worked with Oskar Morgenstern on the Econometrics Research Project in 1959-60. In 1964, he published his results in a book on 'Spectral Analysis of Economic Time Series' (co-authored with his fellow student Hatanaka).
Granger was, however, best known for his concept of cointegration (which he did with Engle) and Granger causality. Co-integration is a term used to describe the genuine relationship between two nonstationary time series. Time series are "cointegrated" when the difference between them is itself stationary. As we know, a stationary time series varies around a common long run average or around a non-random trend. A non-stationary time series, on the other hand, varies around a random trend; in other words, they follow a 'random walk'. The term 'random walk' is borrowed from a metaphor of a drunken man, who is likely to follow no particular direction. Before Granger came along, most economists treated all time-series to be stationary, since there was no method to deal with non-stationary data. This however led to nonsensical results, wherein non-stationary time series showed high correlations, when there was no causality between them (for example, the length of men's trousers could be positively correlated to inflation even though there is no causality between the two). To get over these problems, Granger and Engle suggested that when non-stationary time series are also cointegrated, they have genuine causality. Granger developed econometric techniques to show whether non-stationary time series are co-integrated or not. The Nobel website explains this in terms of the drunken man; if the drunken man is followed at a safe distance by a sober friend, then his path may appear random, but is in fact predictable. To quote:
Short-run changes in time series are frequently stationary, even when the time series themselves are non-stationary in the long run. So, one strategy in the face of nonstationary data was to study only short-run changes. But Granger (working with Engle) realized that such a strategy threw away valuable information. Not all long-run associations between nonstationary time series are nonsense. Suppose that the randomly walking drunk has a faithful (and sober) friend who follows him down the street from a safe distance to make sure he does not injure himself. Because he is following the drunk, the friend, viewed in isolation, also appears to follow a random walk, yet his path is not aimless; it is largely predictable, conditional on knowing where the drunk is — the friend never gets too far away from the drunk, but, on average, stays a constant distance back.
Granger is also well known for his work on causality: in fact, it is famously called the Granger causality. Simply put, Granger causality test is a statistical test to see whether one time series predicts/causes another time series. The current value of a time series is predictable from its own past values. For example, GDP this quarter is imperfectly predicted from information about GDP over the past few years. A second time series is said to 'Granger-cause' another if its past values improve the prediction, one would get just from the past values of the first time series. It is clear that Granger causality is related to cointegration. Granger
and Engle demonstrated that when two variables are cointegrated, then at least one of them must Granger-cause the other. The first important application of Granger-causality to economics appears in a 1972 article by Christopher Sims in which he showed that money Granger-causes nominal GNP, apparently bolstering the monetarist idea that fluctuations in money are the major cause of business cycle.
Granger causality was criticised on the grounds that it was only predictive in a limited way. Granger himself used the term 'temporally related'. He also warned that the test reached 'ridiculous' conclusions if used outside economics.
Conclusion
Engle and Granger were trailblazers in the econometrics of time series. Their work on co-integration and causality (on which they collaborated) and Engle's work on ARCH gave researchers new tools, which made it easier to work on long and varying time series data.
Until the 1980s, most macroeconomic models treated the variables as stationary i.e., the variable fluctuates around a mean value. This is where Granger came along and offered a way to treat non-stationary series. He has shown that macroeconomic models containing nonstationary stochastic variables can be constructed in such a way that the results are both statistically sound and economically meaningful. Granger achieved this by introducing the concept of cointegrated variables, which has radically changed the way empirical models of macroeconomic relationships are formulated today. The second central property of economic time series is volatility. Research on volatility models was initiated by Robert Engle who, in the early 1980s, developed a new concept that he termed autoregressive conditional heteroskedasticity — acronymised ARCH as noted above. Robert Engle has remained at the forefront of research on volatility modelling and made several outstanding contributions in this area for over two decades.
The writer is an IAS officer, working as Principal Resident Commissioner, Government of West Bengal. Views expressed are personal